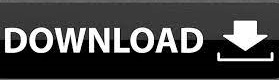
On the other hand, it is not easy to identify whether a specific model design could be generalizable if it is only evaluated on a few datasets. As a result, it needs large amounts of engineering effort to tune the deep learning models in real practice. Moreover, even only considering medical image classification, there are binary/multi-class classification, multi-label classification, and ordinal regression. The biomedical image dataset scales in biomedical image analysis could range from 100 to 100,000. Numerous biomedical imaging modalities are designed for specific purposes by adjusting sensors and imaging protocols. Is deep learning a panacea in this area? Because of the inherent complexity in biomedicine, data modalities, dataset scales and tasks in biomedical image analysis could be highly diverse. ĭeep learning based biomedical image analysis plays an important role in the intersection of artificial intelligence and healthcare 1, 2, 3.
3D BENCHMARK CODE
The data and code are publicly available at. We benchmark several baseline methods on MedMNIST v2, including 2D/3D neural networks and open-source/commercial AutoML tools. The resulting dataset, consisting of 708,069 2D images and 9,998 3D images in total, could support numerous research/educational purposes in biomedical image analysis, computer vision, and machine learning. Covering primary data modalities in biomedical images, MedMNIST v2 is designed to perform classification on lightweight 2D and 3D images with various dataset scales (from 100 to 100,000) and diverse tasks (binary/multi-class, ordinal regression, and multi-label). All images are pre-processed into a small size of 28 × 28 (2D) or 28 × 28 × 28 (3D) with the corresponding classification labels so that no background knowledge is required for users.
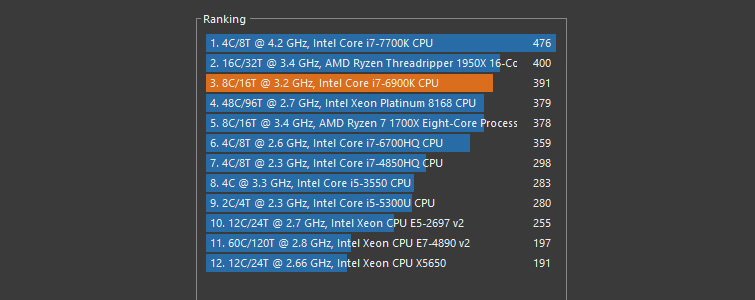
We introduce MedMNIST v2, a large-scale MNIST-like dataset collection of standardized biomedical images, including 12 datasets for 2D and 6 datasets for 3D.
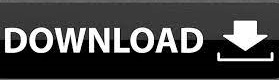